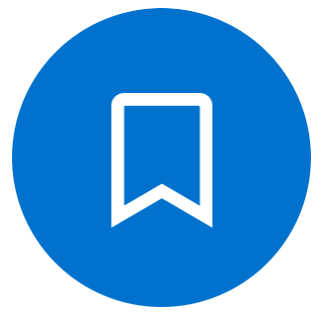
Table of Contents
The following pages are guidelines and best practices concerning generative AI tools and teaching. They will continue to evolve over time based on changes in the technology and use cases in the Johns Hopkins community. Faculty should also consult local divisional guidelines for discipline-specific information if published.
- Background
- Teaching Strategies
- Assessment Strategies
- Guidelines
- Detection Tools: Limitations and Alternatives
- Case Studies
- Resources
- Types of Generative AI Tools for Higher Education
- Using Generative AI Tools to Design Engaging Course Activities
- Using Generative AI Tools to Provide Valuable Assignment Feedback
- Using Zoom AI Companion to Summarize and Organize Recorded Lectures
- Bloomberg School of Public Health – AI In Teaching and Learning
Background
This page was developed collectively by the Johns Hopkins centers for teaching and learning to provide guidance on teaching strategies as they relate to or are impacted by generative artificial intelligence (AI).
What Are Generative AI Tools?
Generative AI tools are powerful technological advancements that have the ability to generate creative content, such as text, images, and even music and video. These tools use advanced algorithms and machine learning techniques to produce new and unique outputs based on patterns and data they have been trained on. The applications of generative AI tools are diverse and have the potential to revolutionize various industries.
In the context of higher education, faculty and instructional support staff can leverage generative AI tools in several beneficial ways. One prominent use case is in content creation and course development. These tools can assist instructional designers and educators in generating engaging and interactive course materials, ranging from automated quizzes and assessments to case studies and customized learning modules. By automating the content creation process, generative AI tools enable educators to focus more on pedagogical strategies and personalized instruction. Furthermore, generative AI tools can enhance the student learning experience by providing adaptive and personalized feedback. They can analyze student responses and generate tailored feedback, highlighting areas of improvement and offering targeted suggestions. This personalized feedback not only supports students in their learning journey but also helps educators identify common misconceptions or knowledge gaps to inform their instructional practices. Another use case for generative AI involves focusing on diversity, equity, inclusion, and belonging (DEIB) to create a more inclusive and equitable learning environment. By generating diverse and representative content, providing language inclusivity, addressing bias, and personalizing learning experiences, generative AI tools can contribute to fostering inclusivity, equity, and belonging for all students.
Although the benefits of generative AI tools in higher education are promising, approaching their implementation with care is necessary. Ethical considerations, such as bias detection and mitigation, should be addressed to ensure fairness and inclusivity. Additionally, striking a balance between automated processes and human intervention is crucial to maintaining the personalized and humanistic aspects of education.
This resource describes various generative AI tools and offer guidance on using their applications for teaching and learning. The best way to learn about and identify potential uses of these tools for instruction is to explore them. Instructors and instructional staff are highly encouraged to work with tools they are considering using before implementing them in their class to identify both their potential and their limitations.
Brian Klaas from the Bloomberg School of Public Health produced several videos providing an overview of generative AI for his Using Generative AI to Improve Public Health course.
- General AI Overview – How Do Large Language Models Work (11:42) (transcript)
- Generative AI Overview (23:39) (transcript)
- Current Approaches, Current Problems (10:18) (transcript)
- Confabulations – Why AI Makes Things Up (8:35) (transcript)
- Generative AI Meeting Assistants from IT@JH
Basic Principles of Using AI-Generated Content
Here are basic principles to consider when using AI-Generated Content
- Selective incorporation instead of full implementation: AI can be powerful in terms of creating large volumes of content quickly and efficiently. However, AI-generated content can lack the creativity and nuance that comes with human-generated content. Understanding the strengths and limitations of AI-generated content to determine when its use is appropriate is essential.
- Augmentation instead of replacement: AI-generated content can be used to supplement or augment human-generated content but should not be used as a replacement for it.
- Validation instead of full acceptance: AI-generated content is not a “set-it-and-forget-it” solution. Continuously evaluating the quality and effectiveness of the content being generated by AI and making adjustments as needed is necessary.
Bias in AI
Despite the possible instructional uses of generative AI technologies, there is an inherent risk of bias in the generated outputs. Biases can be present within the training datasets or the development practices used to create the AI tool. These biases can affect generated content by directly demonstrating prejudice in the content or producing outputs that favor one group or person over another. Academic integrity detection tools are also known to have biases, such as incorrectly flagging writing by students for whom English is not their first-learned language. It is important to acknowledge our responsibility to mitigate the risk of bias generated by AI, as was acknowledged in President Biden’s Safe, Secure, and Trustworthy Development and Use of Artificial Intelligence executive order.
Contributors
Thanks to the following JHU community members for their contribution to this resource.
- Amy Brusini, The Johns Hopkins University Zanvyl Krieger School of Arts and Sciences
- Boris Buia, The Johns Hopkins Bloomberg School of Public Health
- Chadd Cawthon, The Johns Hopkins University School of Nursing
- La Tonya Dyer, The Johns Hopkins University Zanvyl Krieger School of Arts and Sciences
- Lu Chi, The Johns Hopkins Bloomberg School of Public Health
- Pratima Enfield,The Johns Hopkins School of Advanced International Studies
- Jun Fang, The Johns Hopkins University Carey Business School
- Valerie Hartman, The Peabody Institute of The Johns Hopkins University
- Mike Reese, The Johns Hopkins University Zanvyl Krieger School of Arts and Sciences
- Donna Schnupp, The Johns Hopkins University School of Education
- Hong Shaddy, The Johns Hopkins University G.W.C. Whiting School of Engineering
- Amy Whitney, The Johns Hopkins University School of Education
Last updated 3/6/25